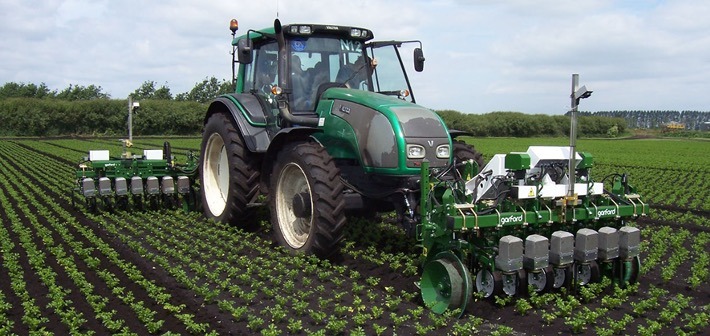
Sensing for targeted weed control
26th August 2020Report from Nick Tillett
Tillett & Hague Technology and Member of BCPC Weeds Working Group
Background
Plant sensing has a long history going back to the 1960s when photocells were used to detect seedings and control sugar beet thinning machines1. Since then sensing technology and the ability to process data has progressed rapidly.
Optical sensors are attractive to crop/weed sensing applications as they do not require physical contact. They come in broadly two categories:
- Non-imaging devices that use colour (visible and non-visible) alone to distinguish live plant material from background, or more ambitiously distinguish between types of plants.
- Cameras that capture digital images in broad colour bands, typically red, green and blue, from which planting patterns can be deduced as well as information about leaf shape and texture.
Sensors can be coupled to mechanisms for real time treatment2 or used to supply data to a mapping system to inform future weed control operations such as patch spraying3.
Commercial state of the art
“Weedseeker” and “WeedIT” are examples of non-imaging sensors that target weeds on uncropped land and pavements. They use a combination of LED illumination and photodetectors to exploit the contrast in reflectance between red and near infra-red in live plant material (Fig.1.). These sensors are housed with fast acting solenoid operated spray nozzles that apply herbicide only when over live plant material. They can be used at normal sprayer speeds, but cost may limit their working width.
Fig 1. WeedIT non-imaging sensor mounted on pavement spraying unit.
Most hoe manufacturers now offer well established camera-based guidance technology to improve the precision and work rate of mechanical weed control. Plant material is distinguished from background on greenness and templates based on prior knowledge of planting pattern are used to estimate crop row or individual plant location. This information is then used to guide blades between rows or around crop plants. Having located crop plants it is also possible to detect and spot treat weeds on the basis that plant material growing between crop plants is weed.
Fig 2. Vision guided mechanical weeder cultivating between and within crop rows
Ongoing research and challenges
Hyperspectral sensors that look at several discrete narrow bands in the electromagnetic spectrum can “finger print” plant species by looking for signature patterns in these bands. Research dating back to the 1990s has shown promise in a lab environment4, but field performance has been disappointing. Challenges include natural light that varies in both intensity and colour, as well as the effects of light transmission and reflection within the canopy. Unfortunately, colour variation between plant species in an agricultural environment is relatively small with chlorophyll being a dominant common factor. Furthermore, those properties change with environmental variables such as moisture stress, nutrient status and plant health.
Cameras with their relatively broad spectral bands are also unable to distinguish between species based on colour alone. However, where differences do exist, they can form limited evidence which can be combined with other information derived from the image to influence a classification. Artificial Intelligence offers the potential to train computers to use a combination of colour, shape and textural data from cameras to distinguish between plant types. This is an active research area5 but is not without its challenges. Leaf shape can change during plant development and with environmental factors and identification is complicated by variable orientation and occlusion. These techniques require high resolution cameras and powerful computers making this approach an expensive one. However, as the cost of technology falls image-based approaches are likely offer the best prospects for species discrimination
Conclusions
Cameras and non-image forming optical sensors are already widely used for weed and crop detection in specialist applications where there is no requirement for species identification. Applications requiring identification of individual weed species are still some way off as there are significant challenges related to variability in the outdoor field environment.
References
- 1966, US Patent 3,533,474 Sugar beet thinner
- Miller and Tillett. 2013, Spot spraying weeds in horticultural crops, AHDB Factsheet 22/13
- Oebel and Gerhards. Site-specific weed control using digital image analysis and georeferenced application maps: On-farm experiences. Precision Agriculture 05, Waganingen Academic Publishers.
- Smith and Blackshaw. 2003, Weed: Crop Discrimination Using Remote Sensing: A Detached Leaf Experiment, Weed Science, Cambridge University Press.
Olsen, A., Konovalov, D.A., Philippa, B. et al. DeepWeeds: A Multiclass Weed Species Image Dataset for Deep Learning. Sci Rep 9, 2058 (2019)